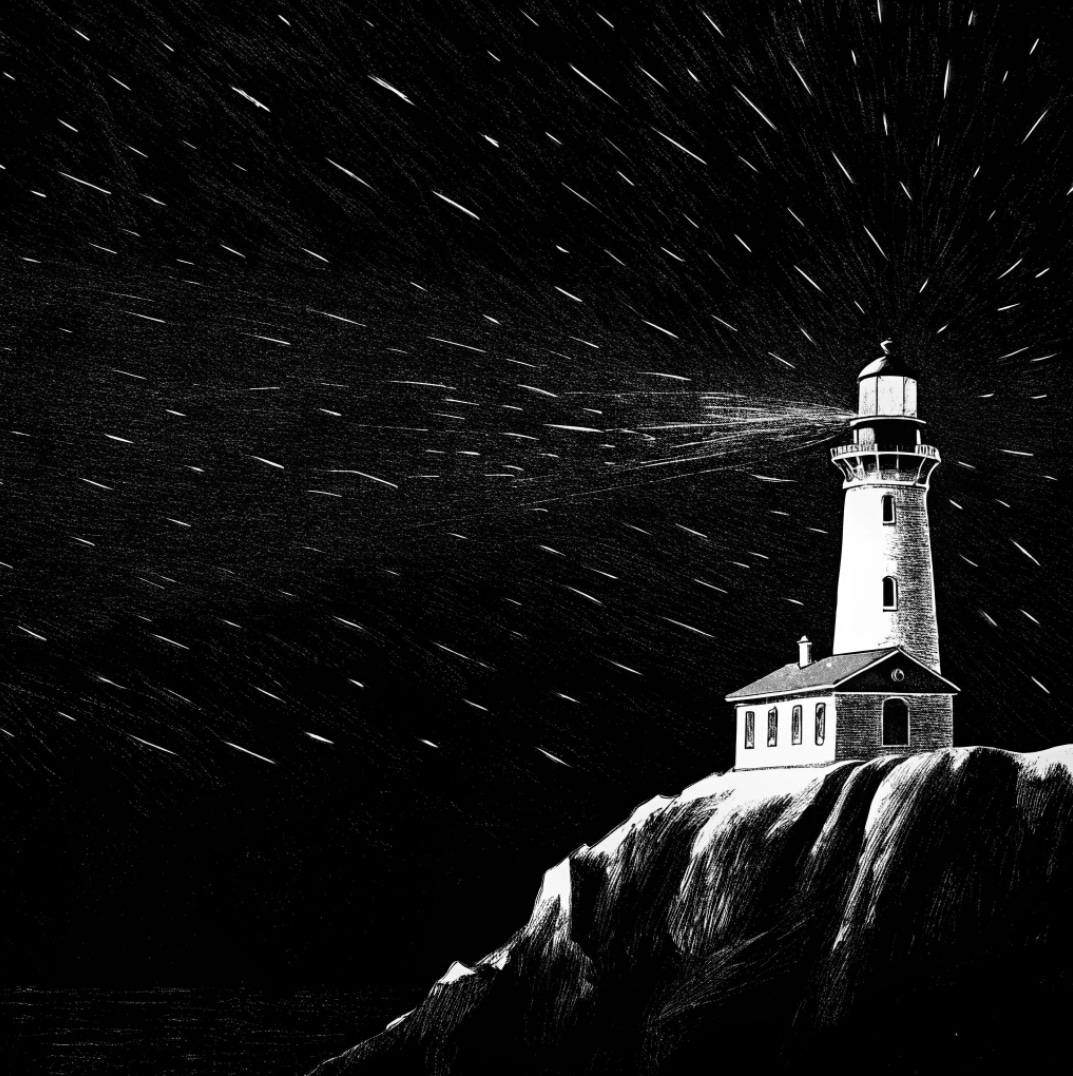
Most discussions about AI capabilities focus on raw intelligence - what tasks the latest models can solve, how they compare to humans, or what architectural improvements might unlock new abilities. But I was overlooking something more basic: what happens when you let these models maintain context over time? This falls into the category that Leopold Aschenbrenner fittingly calls “unhobbling”.
I spent the last few months building what started as a simple solution to a common problem: every conversation with AI starts from scratch. You explain your context, your goals, your constraints - and then do it all again the next day. The obvious fix seemed straightforward: give the LLM some memory of past conversations. But this led to a more interesting question: what exactly should it remember, and how should it use that information?
Here's what surprised me: Given enough continuous context, current models display forms of agency we don't typically see. Not because they've suddenly become more intelligent, but because they can maintain coherent direction across conversations. The system started proactively reminding me about upcoming exams, not when directly asked, but when noticing patterns suggesting preparation gaps. It maintained awareness of deadlines, tracked my study patterns, and intervened at relevant moments.
The technical implementation wasn't particularly complex - just three key components:
- Automated Summaries to maintain context across conversations
- Task Tracking for commitments that come up in discussion
- Prompt Caching to use a lengthy system prompt efficiently
This goes beyond ChatGPT's built-in memory, which stores isolated conversation snippets but lacks the ability to maintain genuine coherence over time. The difference isn't just in quantity of memory, though usefulness scales with quantity to a certain extent.
The interesting challenges weren't technical - they were conceptual. What's worth remembering? How do i create a system prompt that makes Claude use this information effectively?
Most people focus on making LLMs smarter or more capable. But raw intelligence isn't the bottleneck - coherence is. An AI that remembers yesterday's insights is more valuable than one that's marginally better at solving today's problems.
I've open-sourced the system because this problem - maintaining cognitive coherence while working with AI - is worth exploring further. The future will (for now) belong not to those who build the most powerful AI, but to those who figure out how to use existing AI to think more clearly.